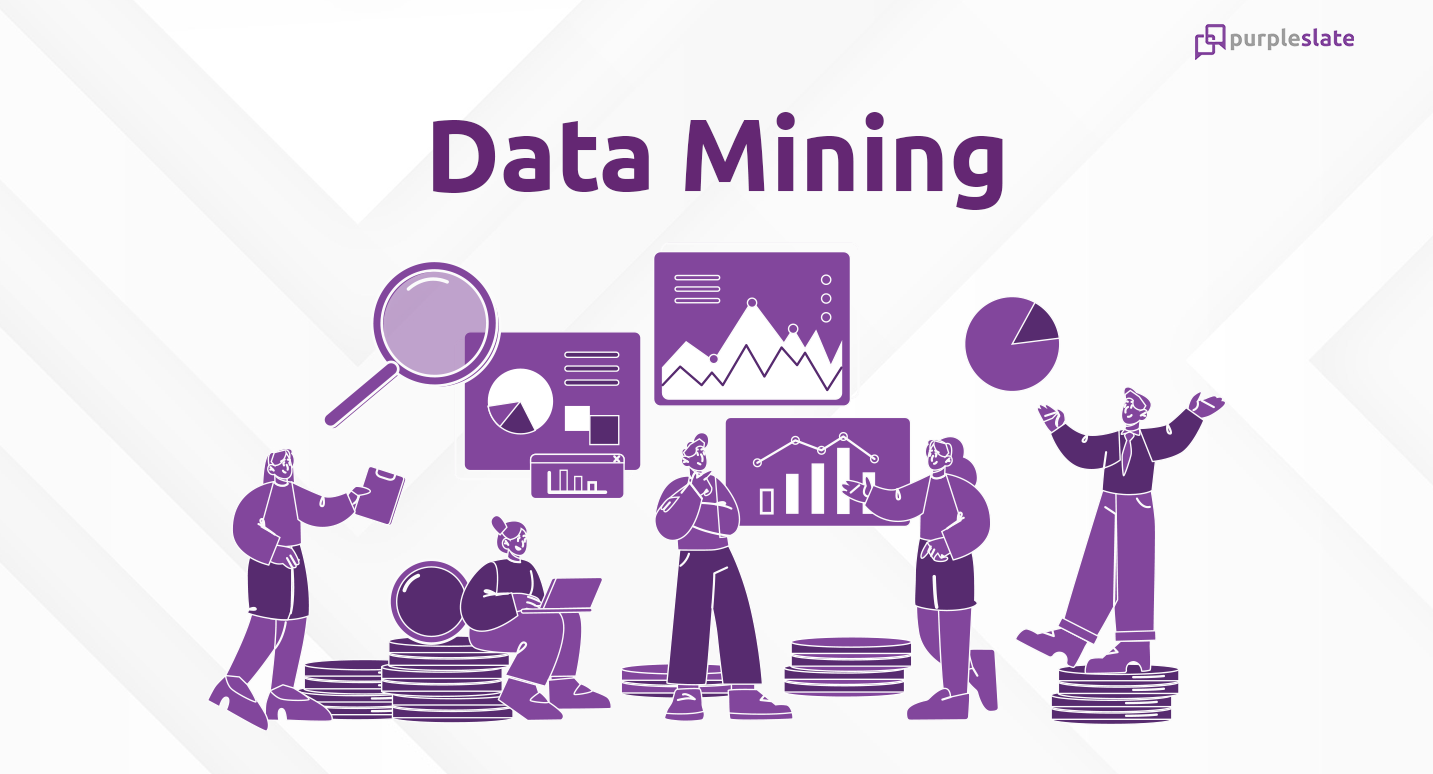
Introduction
We are currently in a data-driven world, rich in information. Knowing there is superfluous and readily available information can feel comforting. However, the more there is available knowledge, the more challenging it becomes for you to get helpful insights. In that case, we will explore data mining aspects. We will discuss data mining, its use, history, how it works, techniques, use cases, and examples. Let’s define data mining and then handle the other techniques and concepts.
What is Data Mining
Data mining is the process of discovering meaningful correlations, patterns and trends by sifting through large amounts of data stored in repositories. Data mining employs pattern recognition technologies, as well as statistical and mathematical techniques – Gartner
It also incorporates searching for wide data sets to establish correlations, anomalies, and patterns that result in actionable information. For instance, weather forecasting requires analysis of historical data to discover patterns and forecast weather conditions depending on climate, time of the years, and other aspects.
The Need for Data Mining
Data mining provides marketers with helpful customer insights about their preferences and behaviors. With such knowledge, they can design targeted advertising and marketing campaigns. Additionally, its results are essential to the sales team in rate enhancement of the lead conversations. Therefore, they can sell more products and services to their clients.
Also, it offers numerous opportunities for entities as it has descriptive and predictive powers. The technology enables the business to forecast the future and make it more profitable. For example, a retail industry can access and analyze past sales patterns and customer behavior which helps in business decision-making.
History of Data Mining
You may be tempted to assume data mining has begun recently since it is often related to new technology. Nevertheless, we are looking at more than a century old rich legacy. The discipline started with Regression Analysis and Bayes’ Theorem used in identifying data patterns.
Increasing technological power and data set complexity has resulted in evolutions of data mining from disks and tapes to massive databases and improved algorithms. By the late 80s, management information system communities, data analysts, and statisticians depended on it.
In the 1990s, mining was identified with the process of Knowledge Discovery in Databases as a step or sub-process. In that case, its popularity grew with the increasing technology and computer capability to store numerous data. Organizations could also store data and process information in computer-readable forms.
Data mining was now a well-understood technique by the end 1990s. An organization could now record customer data and purchases. The mining of the resulting data provided knowledge of clients’ purchasing patterns. Over the last decades, data mining’s popularity has grown continually.
Breaking Down Data Mining Step by Step
Understanding the process will give you a deeper knowledge on its workings.
Collection: Data is gathered, organized, and moved in the data warehouse. It is then stored and managed in the cloud or in-house servers.
Understanding: Data scientists and business analysts examine the data properties and have in-depth analysis from the business’s problem statement perspective. It is addressed through visualization, reporting, and querying.
Preparation: After confirmation of the existing data sources, they are formatted, constructed, and cleaned as desired.
Modeling: Datasets are organized systematically for accurate storage and retrieval from the database.
Evaluation: Based on the business objectives, the results are evaluated, and if there are new patterns, new business requirements are created.
Data Mining Techniques
Businesses can employ various data mining techniques to transform raw data into valuable insights.
Clustering: The clustering technique depends on visual methods of understanding data. This mechanism uses colors and graphs to show data distribution to the various types of metrics.
Sequential Patterns: Sequential pattern technique works on identifying a sequential series of events. The understanding of such patterns is helpful to the organization in recommending other items to customers, boosting its sales.
Prediction: The prediction technique employs current and historical data patterns to forecast the future. In that case, organizations gain helpful insights into future trends that will take place in their data.
The Industries Benefiting from Data Mining
The predictive power of data mining has resulted in significant changes in business strategy designs. In today’s world, organizations can understand the present to make future predictions. Here are a few examples of current industries using data mining:
Telecommunication, Media & Technology: These systems gather and analyze anonymous data from programming, broadcasts, and channel views. Through data mining, networks can create personalized content for television viewers and radio listeners. Also, they can understand their behaviors better and gather information on their activities and interests in real-time. Through determining the relationship between customer aspects like tastes, gender, and age, one can predict their behavior and design personalized campaigns.
Education: Data mining in education is used to categorize and predict teachers’ and students’ performance and dropouts. Educators can keep track of academic progress and enhance their teaching techniques. On the other hand, data mining helps promote effectiveness and efficiency in education management, making it easier for students to select courses.
Banking: Banks can understand market risks better through data mining. Usually, intelligent anti-fraud systems and credit ratings help analyze the client’s financial data, purchasing patterns, card transactions, and other transactions. Through data mining, banks gain a deeper understanding of customers’ online habits or preferences for regulatory compliance obligation management, assess sales channel performances, or optimize marketing campaign returns.
Insurance: Data mining techniques help insurance industries determine future claim amounts in medical coverage and property. It helps them promote effectiveness in planning, preventing fraud activities, and paying incorrect claims.
Manufacturing: Using sensory data, manufacturers can forecast machine failures before they happen. Data mining also helps in identifying commonalities and anomalies in production systems. Therefore, manufacturers can optimize manufacturing capacity and identify patterns that help enhance the quality of all products.
Retail: Data mining helps accurately predict sales volume at particular retail locations to discover the exact inventory levels. It also helps predict future product consumption rates depending on environmental and seasonal conditions. Retail stores can also assess the various product relationships to enhance their layout and maximize sales promotions.
E-Commerce: Data mining is used for up-sells and cross-sells in e-commerce websites to gain more customers. For example, Amazon employs data mining to recommend products based on customer data, enhancing customer experience.
Final Thoughts
Data mining is used across different fields like education, banking, insurance, retail, and manufacturing. When properly used, it offers businesses useful insights into customers and helps develop effective marketing strategies that give them a competitive advantage. Explore the various data mining techniques and employ one of the methods for better decision-making.